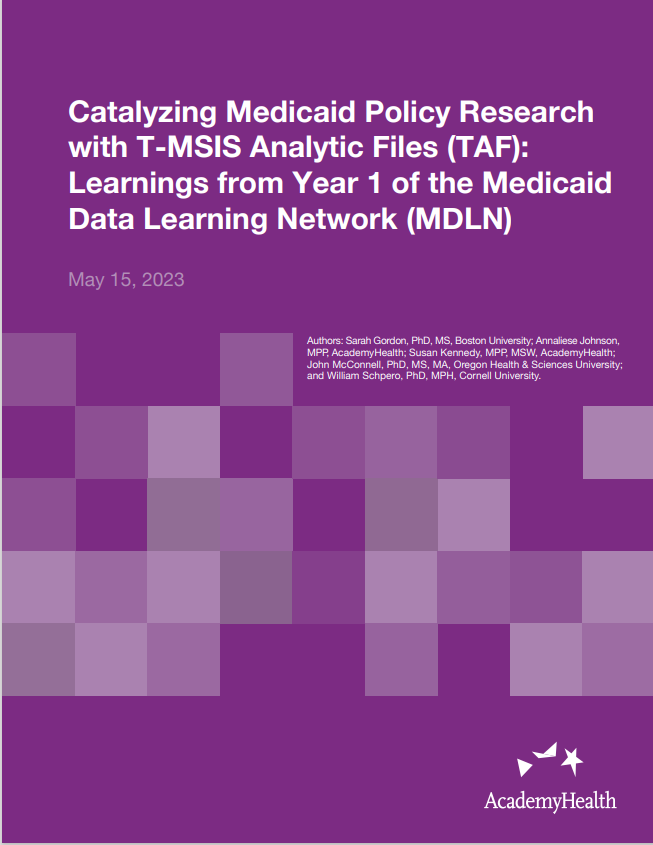
MDLN Year 1 Summary Report
The MDLN's ultimate goal is to improve the quality of the TAF data over time, expand opportunities for health services researchers to use Medicaid claims data, and increase the number of researchers engaged in Medicaid-focused work by fostering peer learning among TAF users. The eight learning sessions from Year One of the MDLN focused on priority topics identified by the MDLN research teams, created space for researchers working with TAF data to share their progress, questions, and solutions in a collaborative environment.
The T-MSIS Analytic Files (TAF) represent a significant improvement in quality and usability over the previous generation of federal Medicaid claims data, the Medicaid Analytic eXtract (MAX), indicating CMS' efforts to enhance the Medicaid Statistical Information System (MSIS). However, TAF remain highly complex. As researchers begin to work with these data, there is an opportunity to share learnings and approaches to avoid duplicative efforts and to distill key methodological standards - enter the Medicaid Data Learning Network (MDLN).
Through a learning series curriculum, the MDLN provides a forum for TAF researchers to share what they have learned using the dataset and to develop consensus on best practices. This report summarizes the substance from the first year of learning sessions, including the key takeaways for both the research and policy communities.
Learning session topics in Year One included:
- Approaches to Standard Measures of Utilization
- Linking MAX and TAF Data
- Navigating Race and Ethnicity Data in TAF
- Methods for Maternal and Reproductive Health Research Using TAF
- Measuring Managed Care Utilization Using Encounter Data in TAF
- Spending in Fee-For-Service and Managed Care Organization Delivery Systems
- Identifying Medications for Opioid Use Disorder in TAF