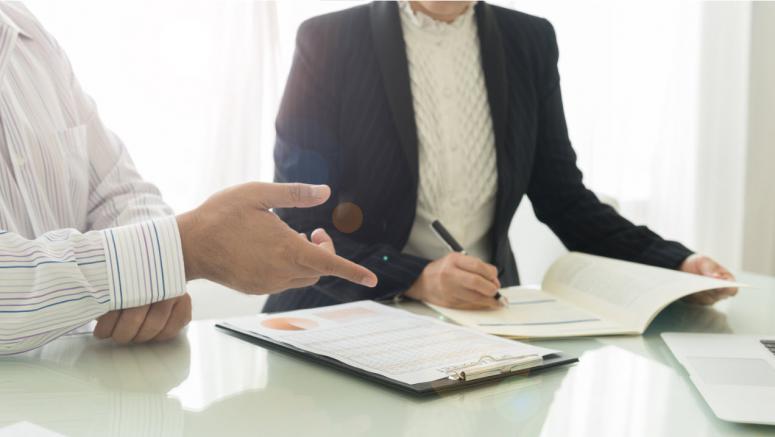
With its patchwork history and volume-based payment roots, the U.S. health care system reflects a tangle of organization, delivery, and payment approaches. We seek and reimburse for care across a decentralized landscape of unevenly incentivized payers, insurers, providers, and other stakeholders. In return, the U.S. consistently (and by large margins) spends more per person than any other country on health care, driven by how much we pay for services (price) as well as how much we use (volume) – this first-in-the-world spending status has only secured middling health results.
Led in large part by the federal government, efforts to tie payment for health care to outcomes and value have grown significantly over the past decade. Since the Affordable Care Act of 2010 (ACA) passed, we have seen a proliferation of government-sponsored efforts to curtail Medicare spending while emphasizing person-focused, appropriate, and high-quality health care. In particular, the Centers for Medicare & Medicaid Services leverages ACA-based authority to launch a wide array of payment and care delivery models intended to nudge health care organizations and individual providers into more efficient, patient-focused, and effective care. In the mix are some sticks, such as penalties to hospitals for excess readmissions, but many more are carrots in the form of financial rewards (shared savings, bonus payments) or seed money. While commercial payers and state Medicaid agencies have followed this lead, system reform is hard work, and change can feel glacially slow. Simply put, it takes time to accumulate robust evidence and we must excavate 50+ years of volume-oriented financial incentives laid by the Medicare program and reorient diverse coalitions of stakeholders that have ossified around the status quo. This doesn’t mean we cannot assess and learn from efforts underway.
So, what works and how do we know?
As we take stock of what the evidence says about which approaches and incentives move the cost and quality needles, and as the promise of data big and small lure us into a sense of transparency and all-knowingness, it is important revisit some fundamentals around interpreting the change we may be seeing.
A handful of considerations from evaluation science can help us be more thoughtful consumers and researchers. Some basic steps you can take, without the aid of an app or fancy wearable, involve asking yourself the following as you ponder a model and weigh the evidence or even interrogate your own research:
- How is this intervention/model/policy supposed to work? Articulating the pathway of effect, and understanding all of the people, places, and things that have to fall into place for the incentive to yield desired impact(s) helps set appropriate expectations and context for understanding and interpreting results. You can even draw a picture of it, which some people call a logic model.
- What data or other information are available to actually observe that pathway you just drew? Where are the information blind spots and what does it mean to not have that information?
- How is success framed? So often, we think about how a policy or intervention impacts spending, utilization, and quality metrics for participating entities relative to some benchmark. However, other dimensions of success can be equally important, such as administration costs, how benefits/unintended consequences might disproportionately accrue to certain communities, or whether the initiative has replicable or generalizable aspects. How do these considerations shape how we talk about reform and transformation?
- Who’s in and who’s out, and what else is going on that might shape what we see? Never underestimate the importance of context! Results may be capturing the effects of an intervention’s “rules of play,” such as how a patient gets counted or excluded from a provider’s spending measures, or how data are collected for performance metrics. The intervention may be inadvertently incentivizing behavior – such as changes in coding practices - that may erroneously suggest the intervention is having an impact. Also, there are so many models and incentives swirling and competing in most markets, that the signal-to-noise ratio often has to be rather high in order to observe clear results and even harder to attribute to specific interventions.
- Do the results make sense, given how the intervention is supposed to work? Statistically significant results are exciting, but p-values are not a cliff, and cannot tell you whether the result is correct or measuring what you think is being measured. It’s important to go back to that logic model and make sure the pathway of effect makes sense in the context of the results. For example, we might observe improved quality under a value-based purchasing model and conclude that the model is having an impact. But, what if we also know that none of the participants have actually changed how they deliver care? How does that change your interpretation of the results?
The allure and promise of data, especially quantitative data, to make sense of how well we are transforming the system is real and important. Expectations and pressures to find effects are also high. As terabytes give way to petabytes and data lakes become data oceans, it is useful to keep these basics in mind, to help us set expectations and context around what the information we have actually can or cannot tell us. Weighing the evidence is not simply a numbers game, we must consider its strengths and its shortcomings, and must always be framed in context.
To hear more on this topic from Lisa and other leading experts, consider registering for AcademyHealth’s Health Data Leadership Institute Sept. 25-26.